Why It’s So Hard to Reform Peer Review
Everywhere you go, you are ranked. Your salary increase is based on performance rankings, as is the likelihood you will purchase another set of earbuds from Amazon. Big data today allows for ranking using algorithms that are constantly foraging through big databases.
Performance goals are invariably numerical. But those who rank others should be aware of the unintended consequences of their announced incentives. Steve Jobs said it well:
“…you have to be very careful of what you incent people to do, because various incentive structures create all sorts of consequences that you can’t anticipate.” (Quoted in Jorge Arango, “Aligning Incentives” from Living in Information: Responsible Design for Digital Places)
Goodhart’s Law, for example, captures the unintended effect of using numerical metrics as goals: “When a measure becomes a target, it ceases to be a good measure.”1
British economist Charles Goodhart’s law applies well beyond his specialty. As a general principle, focusing exclusively on a measurable numerical goal distracts from achieving the broader outcome that the metric is trying to track. Players focus on the numbers and try to game them, which often leads to deception and dishonesty. Crossing the finish line becomes more important than how you got there.
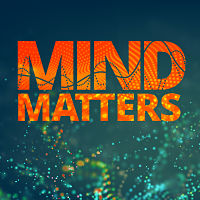
A consequence of Goodhart’s Law is the temptation to cheat to achieve a goal. This is called Campbell’s Law, after an American social scientist, Donald T. Campbell: “The more any quantitative social indicator is used for social decision-making, the more subject it will be to corruption pressures and the more apt it will be to distort and corrupt the social processes it is intended to monitor.”2
In other words, measurement creates a temptation to achieve a measurable goal by less than totally honest means. As in physics, the simple act of measuring invariably disturbs what you are trying to measure.
As a professor at research universities for over 40 years, I have observed that both Goodhart’s Law and Campbell’s Law have had a big impact in higher academia. Much of the impact has been negative.
Here is a historical summary focused specifically on the measures by which professors are promoted and get raises, which illustrates that Goodhart’s and Campbell’s laws are parasites on promotion and tenure metrics.
Publish or Perish!
In the early 20th century, professors were often discouraged from publishing. But then Albert Einstein’s publication of the world’s most famous formula, E=mc 2, led to the atomic bomb, which led to the Allied victory in Japan and the end of World War II in 1945. After WWII, quality soon took a back seat to quantity as federal agencies began to fund universities and research activities began to swell. There was a mandated explosion of scientific publication. The thinking was, if Einstein’s one paper led to the atomic bomb that ended WWII, think what a thousand papers could do!
The phrase “publish or perish” looks to have been coined in 1951 by Marshall “The Medium is the Message” McLuhan (1911–1980), to describe the prevailing academic culture.
University administrators soon began to count publications in promotion and tenure cases. The joke ran, “The Dean can’t read but the Dean can count.” Professors began to write papers using the principle of the “least publishable unit.”3 That is, research content was sliced into papers as thinly as possible, thereby puffing up the publication count on curricula vitae.
Beautifully written, in-depth papers began to disappear from the literature. The law of supply and demand kicked in, resulting in the founding of many substandard for-profit journals. Today, a vast number of journals will publish any paper for a fee.4
Celebration of one’s publication count is ubiquitous. In their short bios appearing at the end of journal papers, some researchers will proudly claim something like “Doctor Procter has over 350 journal publications.” This is like a carpenter proudly announcing “I have pounded 350 nails into boards.” The number of publications or nails is not important. The focus should instead be on what was built.
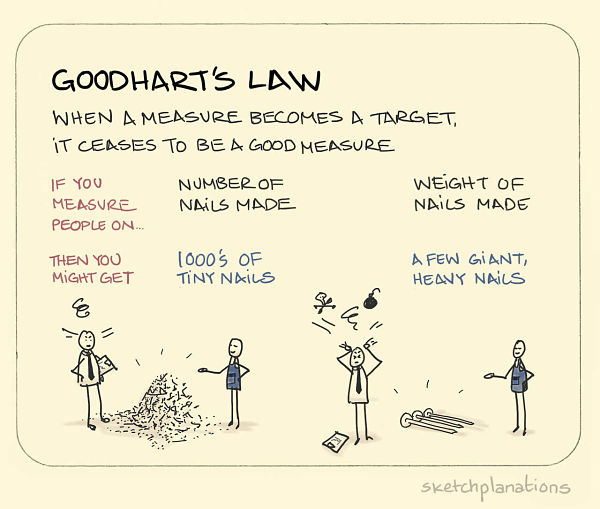
The fact that publication count soon became more important than the quality of the research is an example of Goodhart’s Law. Motivated by quantity over quality, professors became more focused on increasing their publication count than on quality research. John Ioannidis has famously estimated that 90 percent of medical research papers are false. This is why we hear today that coffee causes pancreatic cancer and tomorrow that it is good for you.
In some cases, bad published research results from findings that are not fully vetted by cross-validating data. Getting another paper published is more important than the further vetting provided by additional data. In other cases, there is pure dishonesty on the part of the professor who falsifies data or publishes, as a paper, technobabble written by AI, available on-line.5 This instantiation of Campbell’s Law of dishonesty not only affects those in academia but everyone who reads fake headline news based on the research. Should I enjoy my coffee or not? It’s hard to know what to believe.
In promotion and tenure cases, university administrators should not only count professors’ publication beans but should also taste them. Specialties in an academic department, though, are spread over a wide area and the faculty head count is often so large as to make bean-tasting difficult. The dean who can read and understand the papers of all the faculty members is rare.
The Impact Factor Is Brought to Bear
When Goodhart’s Law makes one metric ineffective, another metric is often substituted. For example, university administrators decided that pure publication count had become ineffective, so they decided to measure the quality of the journals in which their professors published instead. How can that be done? By counting the number of times a journal has been cited by others. The more the journal’s papers are cited in others’ work, the more important the journal is held to be. At least, that is the reasoning. This new measure is called a journal’s impact factor. Important journals like IEEE proudly display their impact factors on their websites.
The impact factor became an important performance metric for journals.6 So then journals began to surrender to Goodhart’s law by gaming their impact factor.7
One distasteful consequence of the adoption of the impact factor is “coerced citation.”8 Here’s an example. I submitted a paper to a journal titled IEEE Transactions on Systems, Man & Cybernetics. My paper was returned without review. I received the following response instead (paraphrased): “Thank you for your submission. Because your paper does not cite any papers in our journal, we are concerned that the material might be outside the journal’s scope and therefore not of interest to our readers.” The associate editor was clearly trying to bolster the impact factor of the journal.
No comment was offered concerning the actual contents of my paper, just the lack of citations to that journal. Content took second place to impact factor—Goodhart’s Law in action! I was invited to resubmit if I could address these concerns. I submitted elsewhere.
In another case, an editor decided in the year’s last issue of his journal to write a review of all the papers published that year, cited in his paper’s references. The impact factor of the journal increased. Goodhart’s Law again. Numerous journals have been slapped on the wrist for playing similar games with their impact factors.9
The H-Factor: Pros and Cons
Apart from the risk of gaming, there is another problem with using a journal’s impact factor to assess the quality of a professor’s publications. Even though a journal might be referenced a good deal, the professor’s paper in that journal may not be referenced at all. Measuring the citations to the professor’s own paper is a different and more accurate metric. Before computerized data analytics, cataloging all of the papers that referenced a given paper was a slow, laborious task. The catalogers had to keep a running tally of who was cited where.
Mined data analytics made finding the citation count a lot easier. Google Scholar does this free for the world to see. But the citation tally for each individual paper is still too much data for lazy administrators to distill. To make things simpler for bean counters, the data can be boiled down to a single number, the h-factor.
The h-factor index was suggested in 2005 by Jorge E. Hirsch, a physicist at the University of California-San Diego, as a tool for ranking the quality of theoretical physicists. 10 If a researcher has a dozen papers cited a dozen times, the researcher has an h-factor of 12. Fifty papers each cited 50 times gives you an h-factor of 50. The greater the h-factor, the greater the researcher. According to Wikipedia,
Hirsch suggested that, for physicists, a value for h of about 12 might be typical for advancement to tenure (associate professor) at major [US] research universities. A value of about 18 could mean a full professorship, 15–20 could mean a fellowship in the American Physical Society, and 45 or higher could mean membership in the United States National Academy of Sciences.
Modern data mining also allows speedy calculation of the h-factor. For researchers, Google Scholar cranks them out. My Google h-factor is about 52. It bounces around by one or two for some reason. That’s a good number to have but some of my colleagues have Google h-factors of over a hundred.
There are many problems with the Google Scholar measure. First, the Google h-factor includes self-citations. If I write a paper and reference one of my own previously published papers, my citation count for the referenced papers goes up. Because a lot of my work is built on my previous research results, I reference myself a lot, which boosts my Google h-factor.11
As with any metric, the h-factor has other serious flaws. Albert Einstein enjoyed a “miracle year” in 1905 when he published four papers. One, on the photoelectric effect, won him the Nobel Prize. Another established the theory behind Brownian motion. A third paper established special relativity and the fourth proposed the most famous equation of all time, E=mc2. If Einstein had been hit by a bus a year later in 1906, his h-factor could never exceed four. Even though each paper has thousands of citations, Einstein would have had only four papers cited four or more times.
Another weakness of the h-factor is that it fails to measure citation quality. Consider “cluster citations” like “The theory of left-hand squeegees has been addressed by many,” followed by 25 citations to the literature. An example of a better “foundational citation” is “Gleason has established an interesting and useful model for the use of left-hand squeegees [citation]. In this paper, we build on this model.” Both cluster citations and foundational citations counted for the same value in the calculation of the h-factor.
Despite these flaws, the h-factor reigns as a metric of academic performance. I once served as a consultant for a major university in choosing their next generation of distinguished professors. “Distinguished Professor” is an honorary title above the full professor title, bestowed on extraordinary researchers.12 In a Skype meeting with members of the awards committee, the h-factor kept coming up. Metrics are fine as a tool for evaluation but they should never be used in isolation. I kept reminding the committee of this fact. We need to taste the beans after counting them. I’m not privy to the final decision concerning the election to distinguished professor at that university but suspect, unfortunately, that Goodhart’s law played a big role.
How the H-Factor Is Gamed
Like any metric, the h-factor can be gamed. A popular unsavory method is reviewer citation coercion: Campbell’s law of dishonesty can kick in when unscrupulous reviewers insist that their papers be cited in order for the paper under review to be accepted for publication. The reviewer wants to increase their citation tally and the author has to pay the ransom of adding the reviewer’s reference to the paper under review. This is blackmail because anonymous paper reviewers wield great power over whether a paper is accepted for publication. Lazy journal editors will blindly enforce reviewers’ comments. Taking time to discern whether the reviewer comment is substantive or spurious is too much of a problem. Since the reviewer is anonymous to the paper author, only the editor in charge knows whether this dishonesty is in play. I know of no editor who monitors such things. Often, authors will do anything asked to get a paper published so they kowtow and include the proposed citations even if they apply only peripherally to the paper’s content.
When I am in charge of reviewing a paper, the author is always given preference in minor difference skirmishes. Good editors should focus on the substance and overall contribution of the paper rather than nitpicky trifles.
I was hit with a reviewer-coerced citation only last month in a paper I submitted to the Journal of the Optical Society of America (JOSA). One review read:
The author provides no reference to recent literature on sub-Nyquist sampling… For example, … work by [suggested citation #1] and [suggested citation #2] [consider] sampling of non-bandlimited signals.
Suggested citation #1 was borderline applicable to my paper and I included it in the revised paper. Suggested citation #2 had nothing to do with the topic of the paper and, I suspect, was a reviewer-coerced citation. I’m at the point in my career where I care little about the timing of paper acceptance. There are always other journals. I’m not up for promotion so I do not suffer from promotion and tenure deadline anxiety. Thus I responded to the editor more boldly than I would have in my younger days. I wrote:
“I trust the editor is monitoring to assure there are no ‘reviewer-coerced citations’ by the reviews. This is an often-practiced conflict of interest. I like it when the reviewer says something like ‘I am not a co-author of any of the papers I suggest.’ This is something I always do when reviewing papers when suggesting additional citation. It’s good business.
I’m unsure whether any action was taken against the reviewer in this matter but I’m happy to report that the JOSA editor read my response and accepted my revision. In this specific case, the reviewer-coerced citation was kicked to the side of the road.
Here’s another way the h-factor can be gamed. I did an experiment a few years back. Google Scholar gives links to papers I post on my website. So I wrote a phony paper and included a few of my previous publications in the references. If I added this phony paper in the same folder as my other papers, would Google Scholar tally the citations in my phony paper in my citation index? The paper was: Ingrid Nybal, Woodrow Tobias Jr, R. J. Marks II, and T. X. Waco. “Evaluating RF Efflorescence Using Event-Driven Models.”
And sure enough, Google discovered the paper and my citation tally went up! My experiment was over so I deleted the paper on my website. But, like an elephant, Google Scholar never forgets. Type “Nybal” and “Tobias” in a Google Scholar search and my phony paper is listed at the top (as of July 18, 2019).
R1 and Beyond…
Goodhart’s law seems to apply to all performance metrics.
My university, Baylor, is on a quest to achieve a top rating from the Carnegie classification of universities. The top ranking, R1, is determined by bean counts like the “the levels of Ph.D. production and external funding.” Does anyone doubt that the pressure to produce more Ph.D.’s will lower the quality of the Ph.D. degree? There are those who say this will not happen because faculty are professional and will not sacrifice quality for a higher Ph.D. count. Goodhart’s law says otherwise.
What’s the answer? How can Goodhart’s Law and Campbell’s Law be mitigated in academia? There is a solution but it requires greater effort than simple bean-counting or coming up with a new metric.
For promotion and tenure, professors are always reviewed by other accomplished researchers in their own fields. These reviews need to be weighed carefully in the decision process. Professors who review are busy and many review letters are hastily written. Many regurgitate bean counts like “Professor Lesser has twelve publications, which is appropriate for someone at his stage in their career.” Rarer is the reference letter that explores in depth the contribution of the candidate under review. External references prepare their reviews out of professional courtesy. They are not paid. Maybe they should be. Even with the in-depth reviews, the effects of cronyism on one hand and professional conflict on the other must be filtered.
Citation quality counts as unsolicited external review. If a paper builds on the research of a faculty member under review, that’s a big deal. But cluster citations mean next to nothing.
It’s a tough and time-consuming job to evaluate the performance of a professor. It’s easiest to just count beans. But, as I’ve been saying, if we want to minimize the undesirable impact of both Goodhart and Campbell’s laws, those beans must be tasted, chewed, swallowed and digested after they are counted. That’s hard work and it requires much more of a commitment to reform.
Notes
1 Here’s the definition from Goodhart’s 2006 paper: “Goodhart’s Law states that ‘any observed statistical regularity will tend to collapse once pressure is placed upon it for control purposes’.” The succinct form quoted above is said to have originated with Marilyn Strathern. See also: The Business Dictionary. (BACK)
2 Donald T. Campbell, “Assessing the impact of planned social change.” Occasional Paper Series 8 (1976). The .pdf of the paper is listed as “pending restoration.” (BACK)
3 Whitney J. Owen, “In Defense of the Least Publishable Unit,” Chronicle of Higher Education,February 9, 2004. (BACK)
4 Here is a list of hundreds of suspected “predatory journals,” as they are called, last updated May 28, 2019. (BACK)
5 Richard Van Noorden, “Publishers withdraw more than 120 gibberish papers”, Nature(February 24, 2014) (BACK)
6 There are similar journal quality metrics such as the journal’s “eigenfactor.” (BACK)
7 John Bohannon, “Hate journal impact factors? New study gives you one more reason,” Science, Jul. 6, 2016. (BACK)
8 Allen W. Wilhite, Eric A. Fong “Coercive Citation in Academic Publishing” Science 03 Feb 2012: Vol. 335, Issue 6068, pp. 542-543 (purchase or subscription required) (BACK)
9 Richard Van Noorden, “Record number of journals banned for boosting impact factor with self-citations,” Nature Newsblog, 29 Jun 2012. (BACK)
10 Hirsch JE. “An index to quantify an individual’s scientific research output.” Proc Natl Acad Sci U S A. 2005;102(46):16569–16572. doi:10.1073/pnas.0507655102 (open access) (BACK)
11 There are other h-factor calculations that exclude self-referencing and even exclude citations from your paper’s co-authors. (BACK)
12 I am a Distinguished Professor. I’m not sure I deserve the title, but I have lower back pain and don’t deserve that either. (BACK)