Impact Looks Different Across Disciplines So Let’s Acknowledge That
Researchers are increasingly requested to demonstrate that their research has produced an impact on society. What do researchers do when they address this request and say “my research has indeed produced an impact”?
They do two important things: they produce historical ‘impact’ statements, and fill their historical statements with causal reasoning. This presents serious challenges for researchers in the social sciences and humanities (SSH), as we have found in our research.
Historical statements are a peculiar type of sentence, because there is no way to control directly for their truth. You can control (to a certain extent) the truth of an experimental statement, by trying to reproduce the initial conditions and the experimental protocol. Even if you don’t actually reproduce the experiment, if you are a scientist you know that, in principle, you might. For this reason a causal statement produced by a scientist is usually accepted by the readers. If a scientist says: A produces B, we tend to believe this causal relation can be corroborated, or disconfirmed.
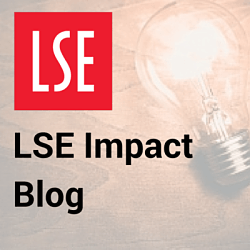
But what about historical statements? What about if you say: A produced B in the past? There is no way to reproduce the conditions. There is no way to control directly. We must believe that you are using the best available knowledge of the past to build up your causal argument. In a word: you must be credible.
And now comes another problem. If a historical statement is made by a scientist, we tend to believe it because it looks similar to something that is done regularly, that we can control. It is credible. If a scientist states: I have discovered a molecule and this molecule has an impact on, say, a new drug, we tend to believe this claim, because people expert in the field may easily control for its validity. If I know that you can control the truth of my statement I have an obvious incentive to tell the truth. This is simply not the same for most researchers and the impacts they have in the Social Sciences and Humanities.
In a recent paper I co-authored alongside Nicola Melluso, Filippo Chiarello and Gualtiero Fantoni, we combined two very distant ideas. To start with, we revisited the debate (going back to Carl Hempel) in the analytic philosophy of history about how historians build causality statements in their narrative. Then we developed a model of credibility of statements about the impact of research in various disciplines, comparing STEM and SSH. Then we have used a technique called Semantic Hypergraphs, recently introduced by Telmo Menezes and Camille Roth, to examine the impact reports of the UK Research Excellence Framework (REF). Semantic Hypergraphs are particularly powerful because they allow the automatic processing of entire sentences in a text, not of isolated words or n-grams.
What did we find?
First, researchers from SSH do not differ from their colleagues in STEM in the effort to build up truly causal statements. They use the same semantic structure, the same set of impact and non-impact verbal expressions. This is understandable: the REF asks them to demonstrate the impact, they try to persuade the evaluators.
But, second, here comes the surprise: researchers use twice the number of words that represent agents of the impact process (see figure). By agents we mean recognizable nominal entities (e.g. physical persons, legal persons, social groups) that have the semantic role of carrying verbal impact expressions, or verbs that imply an impact as a change of state. There are 41,248 agents mentioned in the reports of SSH and only 27,585 in STEM. This means that causal chains in SSH are longer and involve more entities. To demonstrate that your research in, say Archaeology, has produced a social impact you must mobilize a long list of people, from city administrators to government offices, from philanthropic organizations to the media. Or, say, if you want to show that your research in Sociology has produced an impact on the legislation about immigration you must show a long chain of events, linking the circulation of your research to academic reputation, activist organizations, public opinion, political parties, legislative offices, parliamentary bodies. And this is a serious threat to their causal power, because longer causal chains are more fragile, according to analytic philosophers of history. At each node of the argumentation the reader might object that it is not really your research that has done the difference, but something else. Perhaps the same legislation would have occurred anyway without your research etc.

This is not the case for STEM. For engineers, it is easy to see how their patents have been taken up by industrial companies. For medical researchers, we know how preclinical and clinical research is used in the development of new treatments. In other words, the impact pathways of STEM are more standardized and repeatable.
In epistemological terms, scientists in STEM work more frequently with forward causal inference, moving from one cause to its effects, controlling for all conditions (“what happens in a fluid if we increase pressure?”). On the contrary, researchers in SSH work typically the other way round, that is, using backward causal inference (“what was the cause of World War I?” or “how did Picasso came to conceive Guernica?” or “who is the likely author of this unpublished manuscript?”). In these cases there are usually many potential causes, all of which must be identified and examined thoroughly. The conclusions do not have the form of a law-like statements, but of highly circumstantial statements. Do not mistake: this does not mean at all that these statement are less rigorous, they are different.
But, the unpleasant consequence is that the stories told by STEM researchers about the impact of their research are more credible than the stories from SSH. This looks like an unfair advantage. In response to this perception, researchers writing about impact in SSH have developed a few tactics. One is institutionalisation of the impact process (e.g. hearings in official legislative procedures, or formal advisory roles): they place their research into a context that looks similar to the highly structured pathways of their peers in STEM. Another is selectivity: they show that their research has been selected among many competing others, suggesting implicitly that, if the users of research have selected it, it must be valuable by definition. Closely related is repetition: if a researcher is invited over and over again to give seminars, or training sessions, we must assume there is an impact on those who invite him.
But the fundamental issue remains: given that the epistemology of STEM and SSH is not the same, it is wrong to ask researchers to demonstrate an impact in the same way. As research evaluation systems such as the REF are taking place and their results are reflected upon, perhaps we should think again about this implicit assumption.